Overview
We introduce our Graph Convolutional Network (GCN) as a plugin to enhance the estimated 3D poses. Our GCN is trained on the BlendMimic3D dataset, which provides a diverse range of occlusion scenarios. This allows the network to learn and adapt to various occlusion types, refining the pose estimation for occluded joints.
Our graph dynamics model considers six classes as neighboring nodes for each keypoint:
- Center (red)
- Physically-connected node closer to the spine (blue)
- Physically-connected farther from the spine (green)
- Symmetric node (pink)
- Time-forward node (orange)
- Time-backward (yellow)
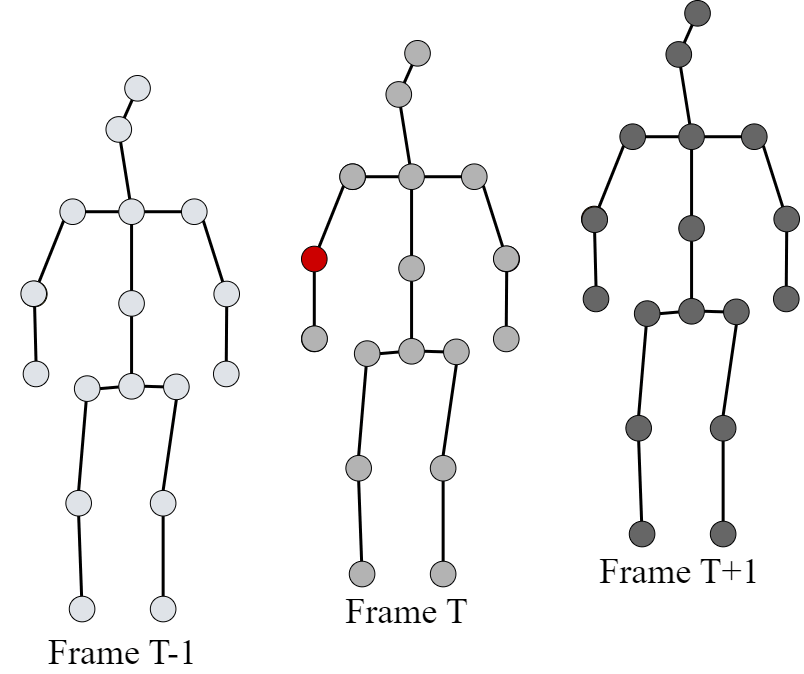
Results
Our results show the significant impact of the GCN block compared to previous methods in occlusion scenarios, as evidenced by the BlendMimic3D results. Below, we provide an overview of both CPN-based and Detectron2-based detections, utilizing the Human3.6M and BlendMimic3D test sets.
Human3.6M (Avg ± σ [mm]) |
BlendMimic3D (Avg ± σ [mm]) |
||||
---|---|---|---|---|---|
Model | 2D HPE | MPJPE | P-MPJPE | MPJPE | P-MPJPE |
VideoPose3D | CPN | 47.8 ± 9.29 | 37.4 ± 7.10 | 175.0 ± 7.20 | 112.0 ± 8.42 |
+ GCN | CPN | 56.3 ± 9.33 | 42.4 ± 7.06 | 112.7 ± 6.76 | 87.2 ± 5.29 |
VideoPose3D | Detectron2 | 57.3 ± 9.96 | 43.6 ± 8.14 | 198.0 ± 7.88 | 122.5 ± 3.67 |
+ GCN | Detectron2 | 59.7 ± 10.35 | 44.1 ± 8.08 | 127.7 ± 11.42 | 95.8 ± 6.90 |
PoseFormerV2 | CPN | 46.0 ± 9.08 | 36.4 ± 7.05 | 148.6 ± 8.00 | 107.7 ± 5.78 |
+ GCN | CPN | 49.6 ± 9.85 | 37.3 ± 7.16 | 107.5 ± 2.03 | 81.6 ± 4.76 |
PoseFormerV2 | Detectron2 | 76.5 ± 19.97 | 55.6 ± 11.97 | 155.0 ± 9.35 | 112.2 ± 7.90 |
+ GCN | Detectron2 | 60.9 ± 11.55 | 44.6 ± 9.29 | 106.9 ± 8.13 | 76.5 ± 7.04 |
D3DP | CPN | 41.4 ± 8.19 | 33.2 ± 6.42 | 100.7 ± 7.94 | 79.0 ± 5.88 |
+ GCN | CPN | 56.2 ± 7.20 | 40.9 ± 6.22 | 95.3 ± 3.58 | 72.1 ± 4.09 |
D3DP | Detectron2 | 51.9 ± 7.79 | 40.3 ± 7.01 | 99.9 ± 11.19 | 79.6 ± 8.08 |
+ GCN | Detectron2 | 58.7 ± 7.75 | 42.1 ± 6.89 | 95.3 ± 4.86 | 74.3 ± 4.50 |